The Importance of Training Data for Self-Driving Cars
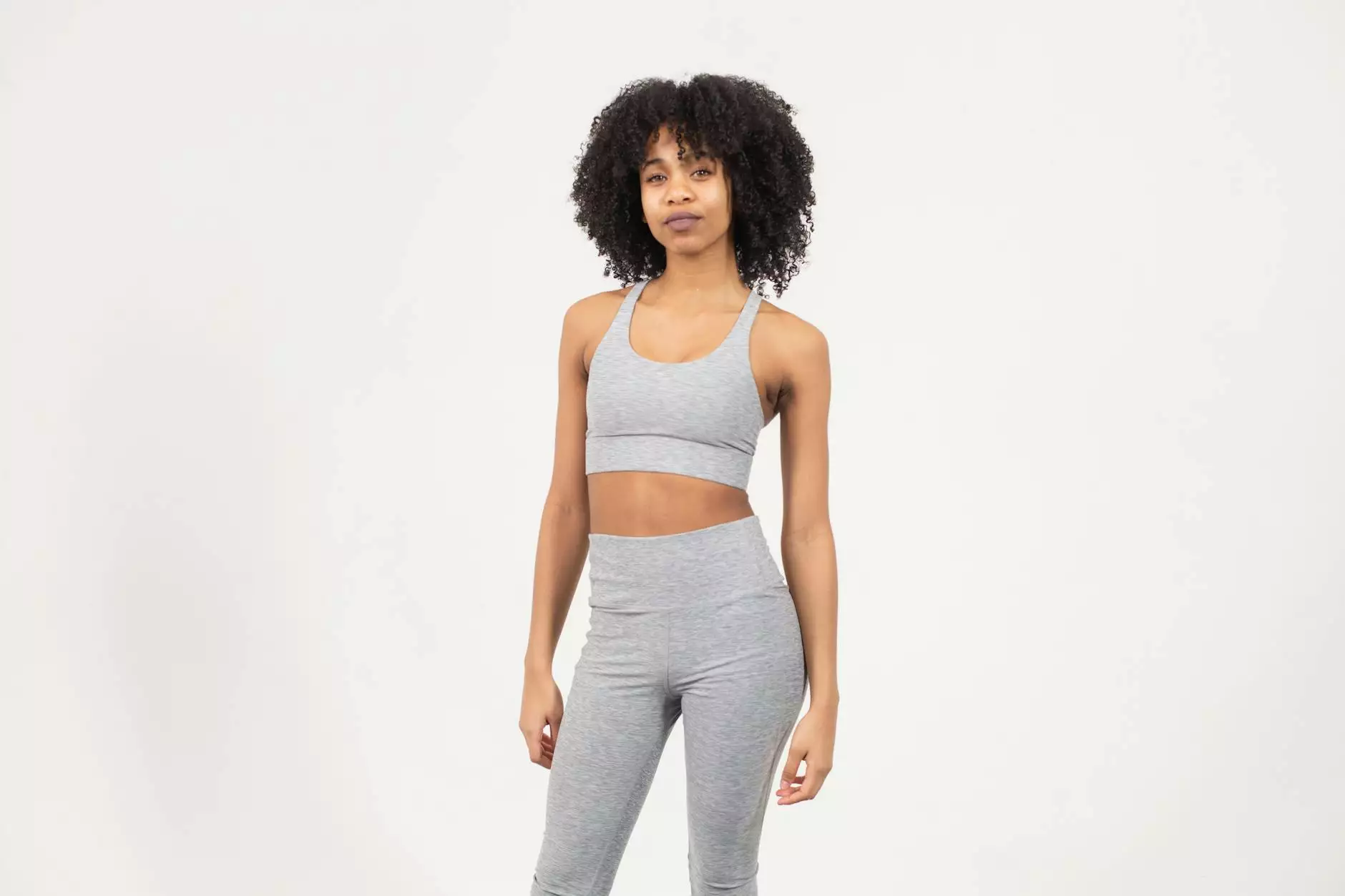
Welcome to keymakr.com, your reliable source for home services within the "Keys & Locksmiths" category. We pride ourselves in delivering high-quality locksmith solutions to meet all your needs. Our expert team of professionals is dedicated to providing exceptional services, ensuring your satisfaction.
Understanding the Role of Training Data in Self-Driving Cars
In this fast-paced technological era, self-driving cars have become a topic of great interest and innovation. However, one might wonder, "How do these vehicles navigate and make accurate decisions on the road?" The answer lies in the critical aspect of training data.
Training data forms the foundation for developing intelligent algorithms that power self-driving cars. It is a collection of various input signals captured during real-world driving scenarios, including images, lidar data, radar data, GPS coordinates, and more. This data is then used to train machine learning models, enabling autonomous vehicles to interpret and respond to their environment accurately.
The Significance of High-Quality Training Data
When it comes to training self-driving cars, the quality of training data plays a vital role. High-quality training data ensures that the algorithms can handle diverse driving conditions and make informed decisions in real-time. Let's explore some key aspects that make training data crucial for the success of autonomous vehicles:
1. Varied and Comprehensive Data
For self-driving cars to navigate safely, they must be exposed to a wide range of scenarios during the training phase. Training data should include situations such as different weather conditions, road types, pedestrian interactions, and complex traffic scenarios. By incorporating a diverse dataset, autonomous vehicles can be better prepared to handle real-world driving challenges.
2. Accurate Annotation and Labeling
Training data must be accurately labeled with appropriate annotations to enable machines to recognize objects and make informed decisions. This involves labeling objects such as vehicles, pedestrians, traffic lights, road signs, and more. Collecting training data with precise annotations ensures the development of robust algorithms capable of real-time object detection and prediction.
3. Real-Time and Dynamic Data Collection
Collecting real-time and dynamic training data is crucial because road conditions and scenarios are constantly evolving. By continuously updating training data, developers can ensure that self-driving cars are equipped with the latest information to handle unforeseen situations. Real-time data collection also assists in improving the overall accuracy and reliability of autonomous driving systems.
4. Safe Data Annotation Environment
The process of annotating training data requires a safe environment where experts meticulously label objects and scenarios. This environment should adhere to strict quality control measures to minimize errors and produce accurate training data. A secure annotation environment ensures that self-driving cars are trained with reliable and trustworthy information.
Conclusion
In summary, the significance of training data cannot be overlooked in the development and success of self-driving cars. High-quality training data, encompassing a wide variety of scenarios, accurate annotations, real-time updates, and a safe annotation environment, paves the way for building reliable autonomous vehicles.
At keymakr.com, we understand the value of training data for self-driving cars and embrace cutting-edge technologies to deliver top-notch locksmith services in the "Keys & Locksmiths" category. Trust us to provide the expertise you need for your locksmith needs while staying ahead in an ever-changing business landscape.
training data for self driving cars