Unlocking Efficiency: The Role of Medical Data Annotation in Healthcare
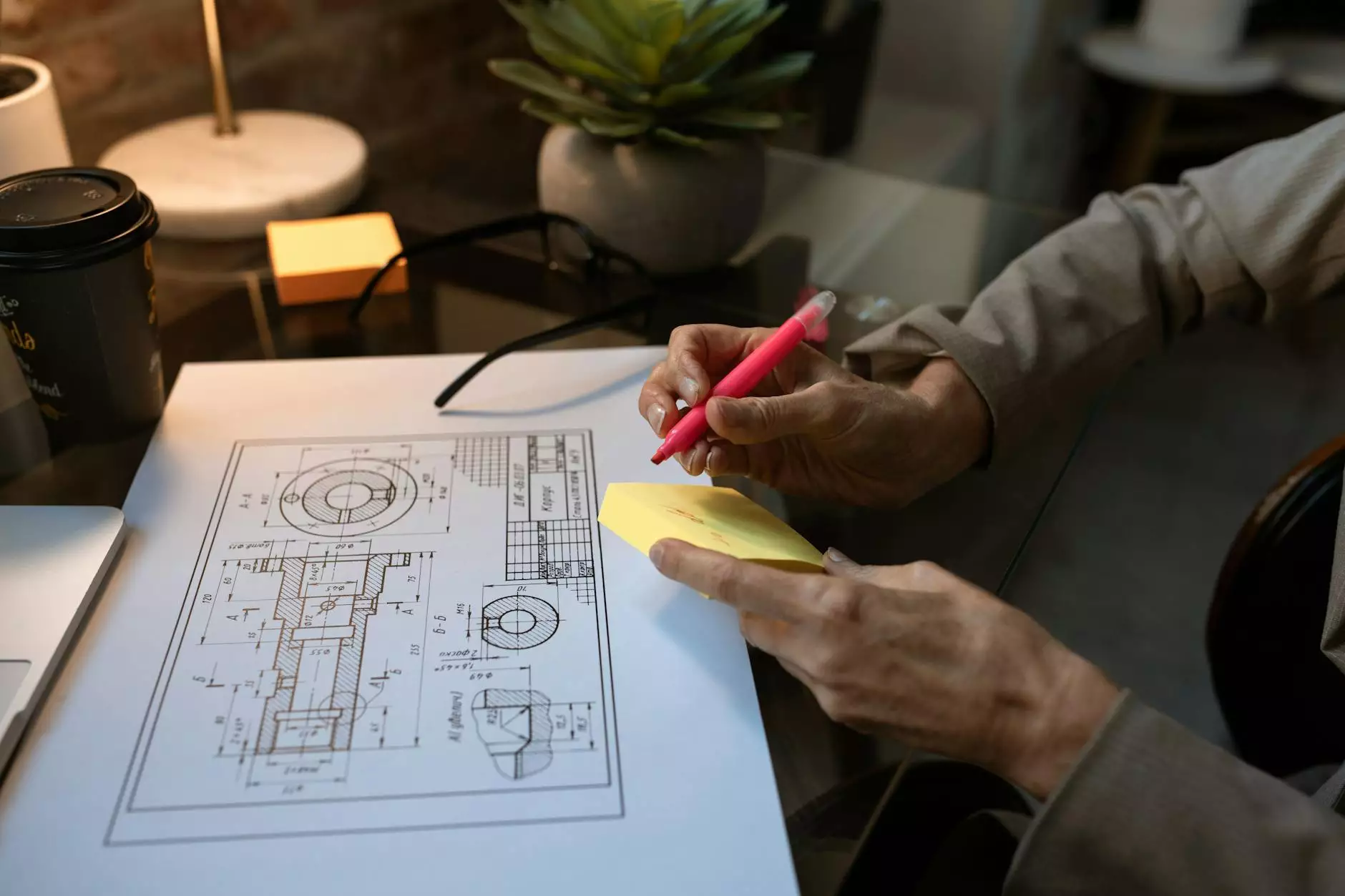
Medical data annotation is a crucial process in the evolving landscape of healthcare, driving improvements in clinical outcomes and facilitating the advancement of medical technologies. In an age where data drives decision-making, the role of annotation cannot be overstated. This article will explore the depths of medical data annotation, its significance, tools, techniques, and best practices, while highlighting how Keymakr, a leader in software development, enhances this vital process.
What is Medical Data Annotation?
Medical data annotation involves the meticulous labeling and classification of medical data, which can include images, text notes, and other health-related information. This process enables machine learning algorithms and artificial intelligence systems to understand and interpret medical data accurately.
The Importance of Medical Data Annotation
As the healthcare industry becomes increasingly data-driven, the demand for accurate and reliable data annotation grows. Here are some vital reasons why medical data annotation is essential:
- Improved Diagnostic Accuracy: Annotated medical images, such as X-rays and MRIs, enable AI systems to identify anomalies more effectively, leading to earlier diagnoses and better patient outcomes.
- Enhanced Research Capabilities: Research studies depend on well-annotated data to derive meaningful insights and make scientific advancements.
- Efficient Training of AI Models: High-quality annotation ensures that AI models learn accurate patterns in data, resulting in robust and reliable healthcare solutions.
- Streamlined Workflows: Properly annotated data improves communication among healthcare professionals, leading to streamlined workflows and reduced overhead costs.
How Medical Data Annotation Works
The medical data annotation process includes several key steps:
1. Data Collection
The first step involves gathering data from various sources, including electronic health records (EHRs), medical imaging systems, and other healthcare databases. This data can encompass anything from radiology images to pathology reports.
2. Data Preparation
Before annotation can occur, the collected data must be organized and formatted correctly. This may involve cleaning data to eliminate errors and ensure consistency across datasets.
3. Annotation
During this stage, human annotators or automated tools assign labels to the data based on predefined criteria. For example, in the case of medical images, radiologists may highlight specific areas of interest or annotate abnormalities detected in the scans.
4. Quality Assurance
Quality checks are critical in the medical data annotation process. This may involve double-checking annotations, conducting inter-annotator reliability tests, and using software tools to ensure accuracy. Without proper quality assurance, the reliability of the dataset may be compromised.
5. Data Usage
Once annotated, the data can be utilized for various applications, including training AI models, conducting clinical research, and improving medical decision-making processes.
Key Tools and Technologies in Medical Data Annotation
Using the right tools for medical data annotation can significantly enhance the efficiency and accuracy of the process. Here are some key tools and platforms commonly used:
1. Annotation Software
- Labelbox: This intuitive platform offers collaborative tools for labeling and managing datasets.
- VGG Image Annotator: An open-source tool primarily used for annotating image data.
- RectLabel: Popular among medical professionals for annotating medical imaging data.
2. AI-Assisted Annotation Tools
AI-assisted tools leverage machine learning to expedite the annotation process by suggesting labels to human annotators, thereby increasing efficiency.
3. Quality Control Tools
Implementing quality control tools ensures that the annotated data meets high accuracy standards and adheres to medical guidelines. Tools that conduct automated screening can detect inconsistencies or errors in the annotations.
Best Practices for Medical Data Annotation
To achieve high-quality results in medical data annotation, consider the following best practices:
1. Define Clear Annotation Guidelines
Develop comprehensive guidelines for annotators that describe specific criteria, labeling conventions, and examples of correct annotations. This will ensure consistency and reduce the likelihood of misinterpretation.
2. Employ Expert Annotators
Utilize qualified medical professionals for annotating medical data, especially in fields requiring specialized knowledge. Their expertise will improve the reliability and accuracy of the annotations.
3. Implement Regular Training Sessions
Conduct periodic training for annotators to keep them updated on new techniques and improve their skills. This investment will pay off with higher quality annotations.
4. Use Version Control
Track changes in the annotations, which allows teams to revert to previous versions if needed. This is particularly important for ongoing projects with evolving requirements.
5. Analyze Feedback
Gather feedback from annotators and users of the annotated data to improve processes continuously. Analyzing this feedback helps identify areas for improvement and leads to enhanced performance over time.
The Future of Medical Data Annotation
The field of medical data annotation is continuously evolving. Here are some potential future trends:
1. Integration of AI and Machine Learning
With the rise of artificial intelligence, the future of medical data annotation is likely to see increased automation. AI tools will assist annotators by providing suggestions, which can ultimately reduce the time and effort required to annotate large datasets.
2. Real-Time Annotation
Advancements in technology may lead to real-time annotation processes, where medical data can be annotated as it is generated, improving the speed at which healthcare professionals can respond to patient needs.
3. Enhanced Data Privacy Measures
As healthcare data becomes increasingly digital, robust privacy protocols will be paramount. Future annotation systems will likely focus on maintaining patient confidentiality while ensuring compliance with regulations like HIPAA.
4. Crowdsourcing Annotation
In some instances, crowdsourcing may emerge as a viable solution for data annotation, particularly for large-scale projects. This involves enlisting a diverse group of individuals to annotate data, with guidance from medical professionals to ensure accuracy.
Conclusion
In conclusion, medical data annotation stands at the forefront of healthcare innovation. As the industry shifts towards data-driven methodologies, the role of high-quality data annotation becomes increasingly significant. Companies like Keymakr are setting the stage for improved healthcare outcomes through advanced software development that supports accurate and efficient annotation processes. Emphasizing best practices, embracing technological advancements, and maintaining stringent quality controls will ensure that medical data annotation continues to play a pivotal role in enhancing patient care and driving medical research.
Investing in high-quality medical data annotation not only improves the effectiveness of healthcare solutions but also fosters better patient experiences, ultimately leading to a stronger and more resilient healthcare system. As we look towards the future, embracing innovations in data annotation will be a necessary step in transforming healthcare capabilities around the world.